January 11, 2019
Top 5 Mistakes of Interpreting a Digital Marketing Experiment
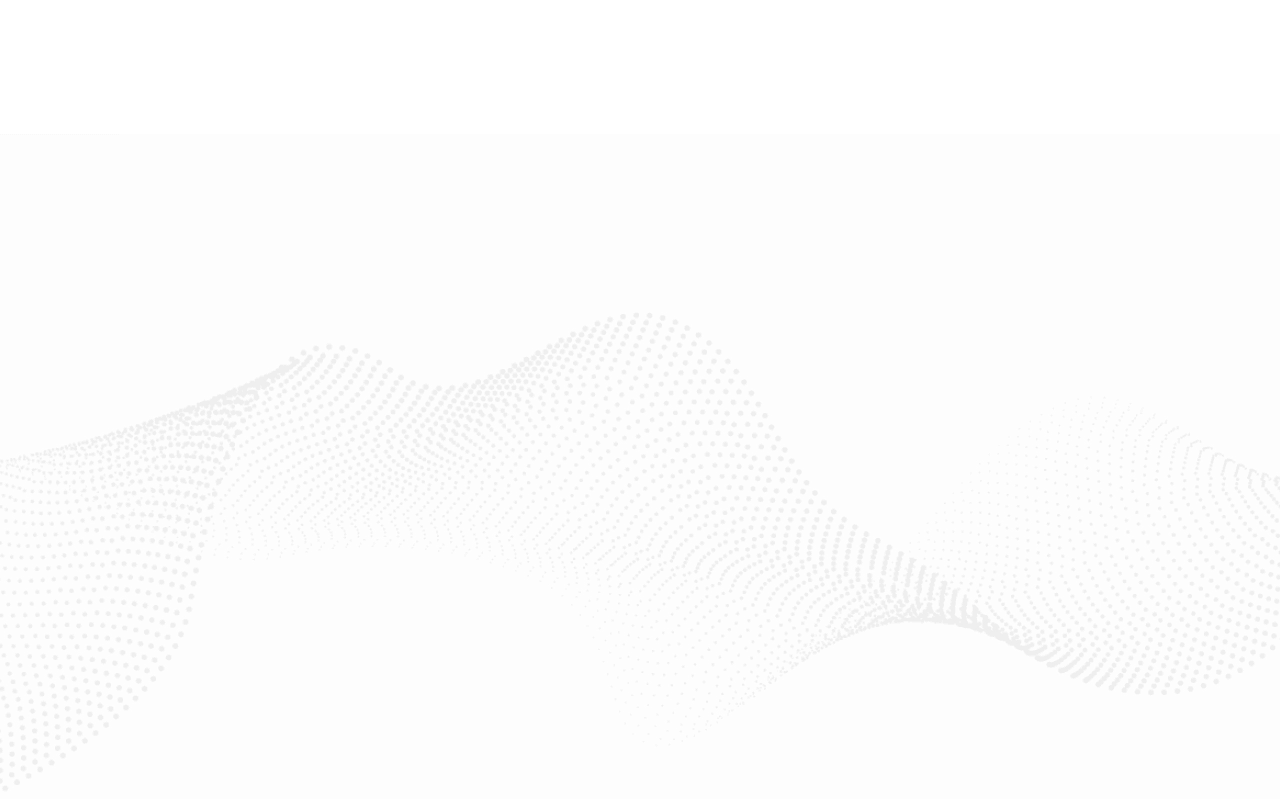
Experimentation is crucial in marketing, and yet so many teams continue to take detours that lead to incorrect conclusions, misinterpret the data, or fail to get the most out of results. In some cases, marketers may use these conclusions to make decisions on words and creative that will not best engage their consumers. To help you avoid falling into the trap, I’m going to walk you through common mistakes marketers make when interpreting experiments.
Extrapolating patterns from a single data point
Although data from a single test is informative, making big updates to your communication strategy based on one result is not recommended. That said, we can take a ton of learnings from experiments, especially when using the multivariant methodology (determining which combination of variations performs the best out of all possible combinations). But even this can still provide only a small glimpse into how the audience behaves. To truly begin to understand what makes customers act, it is best to combine learnings from multiple experiments so that patterns emerge.
Not making a clear distinction between the quality of data
There are three categories of learnings:
Observations: Saying that A performed better than B is an observation, and it doesn’t mean much unless you can prove it with data.
Directional: What do you do when the data seems meaningful, but you have not achieved statistical significance? That type of data can still be used but with less weight attached to it. This is especially true for identifying patterns. For example, you may have a directional insight that having a beach in the image outperforms city motives as it had the best performance in the past 4 out of 5 experiments. The results may not be statistically significant, but such observation can help guide future experimentation and help develop new best practices for creative development.
Statistically significant: The idea of a statically significant result is the holy grail of all statistical models. Whether it is set at 99%, 95% or even 90%, this data is the most important for making critical discussions. A rigorous statistical analysis makes sure that the observed performance difference is not a result of noise, but is indeed the expected relative performance of the tested elements. Note that even with high confidence, typically results just show that A performed better than B. Relative performance gap, or lift, requires additional calculations.
Focusing only on data that fits the narrative or a preconceived notion
We test because we are not sure. In some cases, we mentally map out how the results will look and selectively focus on the data that supports that vision even in cases where other findings are more meaningful for the business. Often, it is a tunnel vision towards the imagery. Although it is important to get that part right, we often spend a disproportional amount of time on images and ignoring other valuable insights produced by a test.
Not planning to build in the learnings into the future experimentation plans
Experimenting with no real plan for how to use the learnings is basically testing for the sake of testing. Something meaningful should change after an experiment is complete, such as the team will use the top creative to generate a better return on ad spend (ROAS) or update best practice for using copy. After all, learnings are only useful if they are used to impact the business in a meaningful way.
Failing to combine the learnings with results from previous experiments
One of the many huge benefits of AI is that it allows us to look at patterns within a large set of data. This is how Persado is able to deliver improved performance to our clients consistently. Teams not yet outfitted with an AI solution can start spotting patterns by reviewing the results of tests done across the company. It won’t give the same robust insights, as AI can look at thousands of combinations quickly, but it’s a good launching point. Did the email team find it is best to use language that evokes Safety (to eliminate worries or doubts; to make one feel secure) in their subject line? Why not try something like “We’ve got you covered: The coziest winter coats of the year” on Facebook? Is mentioning competitors outperforming in some tests, but not in others? Do the images that tend to beat other images have something in common? These observations can help map out a marketing communication strategy and form theories for future tests.