December 22, 2023
Primer on Generative AI for Text in Marketing
By Liza Colburn
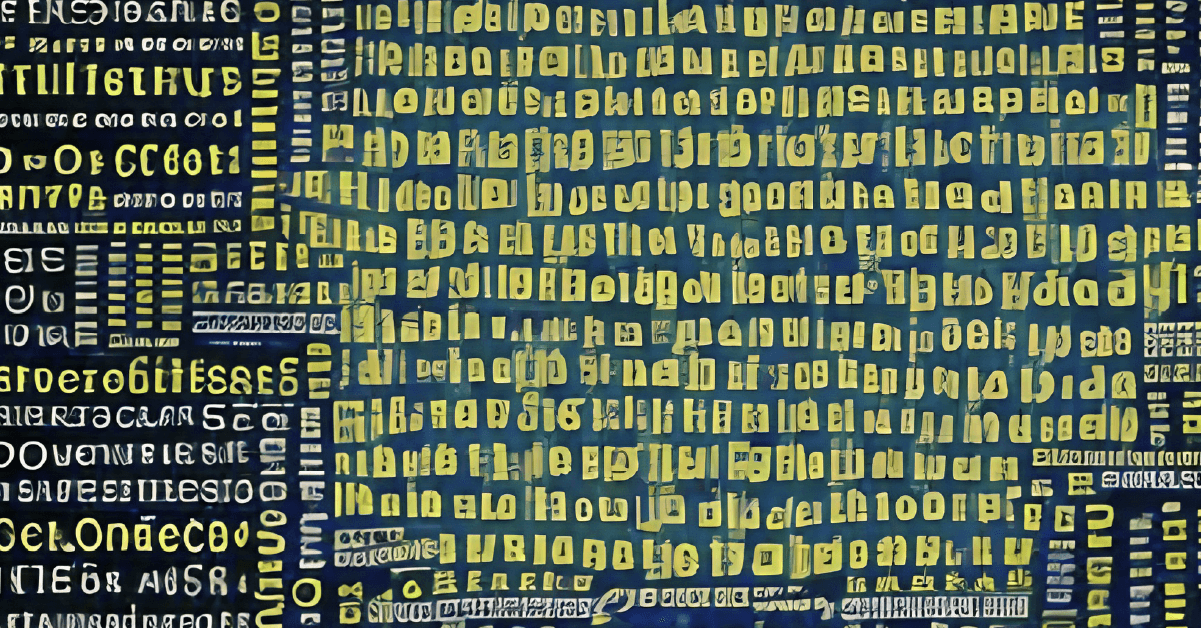
Generative AI (artificial intelligence) for text, images, and videos has risen to the top of the business toolkit. There has been an evolutionary explosion of interest in Generative AI for text in the enterprise. This is happening even as a range of other front- and back-end use cases continue to emerge. We talked about how marketing leaders should think about defining their business goals and selecting GenAI providers in our CMO’s Guide to Achieving Impact with Generative AI. In this blog, we provide a primer on Generative AI for text generation, how it works, and the opportunities and risks it brings to enterprises, particularly for marketing and other customer-facing functions.
What is Generative AI for text?
Generative AI is a form of artificial intelligence capable of creating new text, images, video assets, or voice files in response to “prompts,” or instructions written by a human user.
Generative AI relies on large language models (LLMs) that have been trained on volumes of “language data”. This data includes written text or audio files that train the AI to understand the mechanics of human speech. The field of AI in which LLMs fall is natural language processing.
With LLMs as the foundation, Generative AI solutions often include an application layer that adds specific functionality. For example, Generative AI for text leverages the AI model’s knowledge of human language patterns to create fluent, human-sounding outputs. (This is also part of what Persado does.)
Generative AI for images, in contrast, associates words with their physical equivalents (dog, rose, supernova) and with images of those equivalents, including photos, paintings, or drawings. The AI can then generate new images based on natural language prompts coupled with image recognition or computer vision capabilities.
The evolution of LLMs and Generative AI
Generative AI gained mainstream attention beginning in November 2022 with the launch of ChatGPT. In 2023 alone, around $640 million in venture funding went to startups building solutions focused on Generative AI for text. Enterprises invested another estimated $2.5 billion in Generative AI, which Menlo Ventures points out is a fraction of total AI spend.
Despite the recent upsurge in investments, this technology is not entirely new. Natural language processing has had a place in modern businesses for decades. Amtrak, for example, introduced its voice assistant, Julie, in 2001. One of the catalysts for the explosion of natural language AI as we know it today was the discovery in the 2010s by Google researchers of a technique that led to “Generative Pre-trained Transformers” (or GPTs).
Transformer models are a type of neural network—which are themselves a branch of machine learning. Neural networks mimic the thought patterns of the human brain. These models have an architecture that allows them to evaluate words both individually and in the context of a sequence, as in a sentence. This allows transformer-based language models to quickly ingest and process huge volumes of training data, making language model training much faster and more accurate.
From the transformer innovation to large language models
Developers began to quickly leverage the vast corpus of language data housed on the Internet—among other sources—to train transformers and create the general-purpose LLMs (general purpose because they are trained to generate text for any purpose, not to apply to specific use cases). In response to prompts, the LLMs build sentences and paragraphs based on the most common combination of associated words or phrases.
Since 2021, when the first commercially, general-purpose LLM became available, there are now many. They include Claude by Anthropic, Google’s BERT model, Meta’s LLaMA, GPT-4 by OpenAI, and others. These models have a powerful predictive capacity that enable them to generate content at scale. In multiple enterprise surveys, more than half of respondents are already integrating Generative AI into their workflows.
The risks of using Generative AI
For all their power, the general-purpose Generative AI models listed above are new and untested at enterprise scale. Not all GenAI providers are untested in this way. Persado, for example, has been in business for more than 10 years and runs on a proprietary and market-tested language model. As for the new, general-purpose LLMs, they comes with risks, such as:
Privacy risk from storing and managing AI-generated content.
Enterprises that build or fine-tune LLMs must ensure that personally identifiable information (PII) does not remain embedded in the language models. Removing PII from these models must be done to comply with privacy laws.
Unconscious bias as a result of the data used to train their models.
This is not unique to GenAI but exists across the world of machine learning. Finding large enough datasets to train AI has long been a challenge. Ensuring that the data does not perpetuate harmful stereotypes or otherwise disadvantage certain groups may be more difficult.
Inaccurate information (unintentional).
Generative AI for text has a tendency to make things up, or “hallucinate”. This happens because the AI does not know the difference between truth and lies—it is merely compiling words and phrases into meaningful sentences. Those sentences can contain falsehoods which can damage a brand if not caught and corrected. Meta provides a cautionary tale of the negative impact of AI hallucinations. In 2022, the Facebook and Instagram parent company launched Galactica, an LLM for science that it reportedly developed as a research project. After three days, Meta pulled down Galactica because early users found that it generated text riddled with scientific falsehoods and inaccuracies. Delivered with the authoritative tone of the best scientific writing, it even attributed false findings to real-world researchers. To Meta’s credit, it gained many insights from the experience, which it baked into the launch of Llama and Llama 2.
Inaccurate information (intentional—as with fraud).
The ability for anyone to generate content at scale means malicious actors can leverage Generative AI to create more content for scams. One example is deepfakes, the technology for which is quickly evolving and becoming more difficult to detect. Using Generative AI, bad actors can create videos with voiceovers that look like they are coming from a person who did not, in fact, give their consent. Public figures—like your CEO—and celebrities—like your influencers—are easy targets.
Spam content farms.
These are websites developed to drive search engine optimization and different types of tagging. Programmed using basic AI, these sites disseminate unreliable content and pull traffic from valid brands. Over time, they may also add to the data storage and validity issues highlighted above.
Regulatory uncertainty.
More questions have emerged about the language used to train LLMs and who owns it. Writers and other creators have filed a number of lawsuits in the past year alleging that the LLMs were trained on copyrighted materials that organizations like OpenAI, Midjourney, Stable Diffusion, and others had no right to use. These and other issues are why 77 percent of executives told KPMG that regulation is affecting their AI adoption.
Mitigating risks from GenAI
Technology providers are already taking steps to mitigate these risks. Some of their approaches aim to take advantage of other forms of AI technology. For example, Intel’s FakeCatcher is a real-time deepfake detector that compares one frame to another to determine whether the skin tone has changed from blood pumping under the skin. No blood means it’s fake.
Some of the risks of using general-purpose Generative AI for text derive from the way these platforms work. Because they are designed to predict the next likely word to appear in a sentence, their strength is volume, not quality, accuracy, or context awareness. Alone, general-purpose GenAI will not (and cannot) adapt the text to the reader or the medium. To do that, data scientists must “fine tune” a language model—the technical term describing one approach to refining the training data and adding capabilities to improve accuracy and specificity for a specific use case.
Alternatively, a language model could from the outset leverage training data that is fit for purpose. Persado began training its proprietary language model more than a decade ago using enterprise language that performed well in Fortune 500 marketing campaigns. That’s just one way we ensure that our Motivation AI Platform fits the needs of our customer and doesn’t have the risks noted above.
5 customer-centric business use cases for Generative AI
The possibilities for Generative AI for text are vast. Organizations are already leveraging Generative AI for five common use cases that will change how we work.
Use case #1: Generative AI for text and language in marketing
Using Generative AI for text can help marketers produce much more content than they could on their own. Based on a single campaign concept a marketer could use GenAI to write 20-50 variations of the same call to action for an email or text campaign. She could also plug an initial statement into an AI platform and ask it to create dozens of variations. In this way, Generative AI frees up human time for more demanding work.
Persado, for example, is an enterprise Generative AI for text solution that focuses on optimizing customer engagement. Customers in retail, financial service, travel, telecom, and other sectors are already leveraging the Persado Motivation AI to customize language for acquisition campaigns, customer service, payments, loyalty, and other use cases. What sets Persado apart is the ability to tap into customer motivation to improve marketing performance (i.e. conversions). We do this without the risks noted above. Motivation AI is also different from tools like ChatGPT because the model understands which words and phrases motivate customers to take action.
Persado works with retailers like Kate Spade and Marks & Spencer, financial institutions like JPMorgan Chase and LendingClub, and travel and hospitality firms such as airlines and hotels to personalize customer messages. Across email, text, social channels, and other areas of engagement, these brands are driving incremental revenue, loyalty, and customer engagement.
Use case #2: Chatbots for improving customer service
Digital customer service agents use Generative AI for text to create a more natural conversational experience. The technology is evolving so that customers can write almost anything into a chatbot and receive relevant and helpful responses. According to a report by Gartner, chatbots will emerge as the primary customer service channel for almost a quarter of organizations by 2027.
That comes as no surprise, given that chatbots can instantly respond to customers 24/7 across a variety of channels. Brands can provide better customer service, personalize the experience, and diminish missed opportunities.
The Persado Motivation AI chatbot
Chatbots themselves have many use cases. For example, they can serve as AI-enabled personal shopping assistants, providing targeted recommendations and other suggestions. As a result, chatbots are transforming from their origins as basic customer service tools into a central component in conversational marketing.
In fintech, chatbots can resolve problems in real time using Generative AI.
Use case #3: Improving the customer experience
The rise of chatbots is a supplement, not a replacement for, human interventions in customer service and the customer experience. Generative AI can also improve the customer experience by helping human reps. Examples include:
- Scanning for sentiment insights across all customer contact channels.
- Interpreting needs so as to deliver solutions or steer customers to the right agent the first time.
- Automatically escalating complex or high-value cases.
- Providing targeted personalization for products and services.
Because GenAI can quickly read language, it can capture key in-the-moment details about a customer and trigger pre-drafted scripts agents can follow to solve problems or enable upsell opportunities.
GenAI may also play a role in fully automating superior customer experiences. For example, Airbnb is incorporating it into its platform to uncover potential matches for a customer. To make those matches, Airbnb analyzes a wide range of factors, including price preferences, previous stays, and trip duration. By presenting listings for a variety of locales, both sides of Airbnb’s lodging ecosystem benefit.
Use case #4: Synthetic video and audio
Several companies are developing technology that allows brands to convert written text into words spoken by a synthetic voice. These synthetic voices can switch cadence and inflection based upon the text. AI engineers can even train text models to highlight specific inflection points.
Advances in synthetic video and audio will transform the production of videos that include voice-delivered text. Businesses could leverage this technology to create video advertising, product how-to’s, thought leadership content—the use cases are only limited by the imagination. Dozens of new companies are already offering solutions in this space. Organizations using them can produce more video for less money and time.
As important is the way these advances can make videos more engaging and easier to edit. Updates become easier to do without retaping a human speaker. Customization is also easier. For example, a narrator can have a British accent or a southern US accent to better connect with specific audiences.
YouTube is one example of how Generative AI for text is changing the video creation process. The video site is making GenAI tools available to its creators on the platform. Dream Screen, a new feature, produces AI-generated videos and photos that can be placed in video backgrounds. An AI component makes dubbing YouTube videos into other languages easy.
Use case #5: Generating synthetic customer data
Synthetic data is becoming an increasingly popular way to increase privacy by creating data sources that have no connection to real people. In theory, data scientists could construct these datasets without patterns of discrimination or bias and then use them to train language models. JPMorgan Chase and American Express, for example, are building synthetic datasets to train AI to detect fraud.
The takeaway
The transformation triggered by Generative for text is just beginning. The past year saw a ramping up of interest and increases in investment. That will continue as organizations make strategic decisions about the deployment models and use cases that will deliver value.
For marketers, Persado is here to help. You have options for how to work with Persado. Our self-serve GenAI solution Essential Motivation helps email marketers craft high-performing email subject lines and body copy. Enterprises can also leverage personalized language generation with Dynamic Motivation. One key use case is to especially help reduce online cart abandonment and increase revenue.
Reach out today to schedule a risk free trial to learn first hand the power of Generative AI for text to optimize marketing performance.