January 11, 2024
Risks From AI Hallucinations and How to Avoid Them
By Liza Colburn
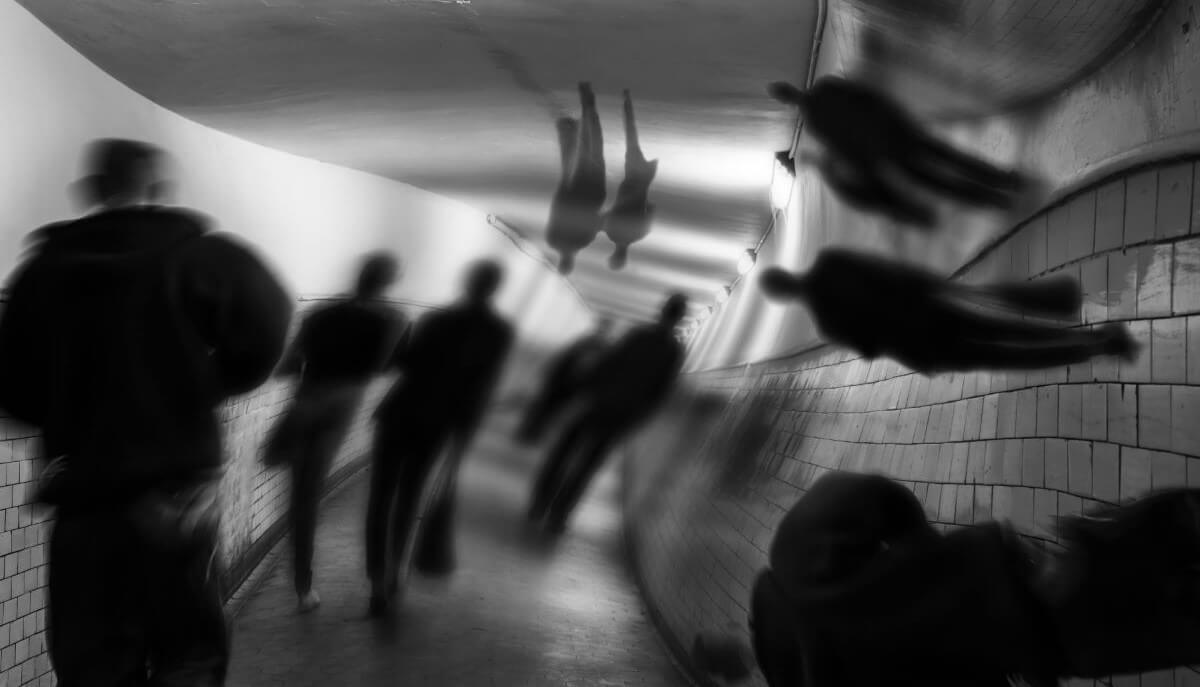
Before the advent of Generative AI, hallucinations were the stuff of fever dreams or chemical substances. More recently, the term has come to refer to the fanciful responses that language models occasionally produce. Alternatively funny and alarming, AI hallucinations raise concerns about the potential risks enterprises can face from leveraging Generative AI. With this article we explain what AI hallucinations are and why they occur, and how enterprises can avoid them.
What are AI hallucinations?
AI hallucinations are AI-generated statements that do not exist in the model’s training data. As a result, hallucinations can include falsehoods that the Generative AI presents as if they were true. AI hallucinations are particularly dangerous for the way they can blend invented information with facts, dates, and real people. The result can be inaccurate and misleading text that is nonetheless compelling and convincing.
For example, when The New York Times (NYT) asked a number of AI chatbots when the paper first reported on AI, each answered with a different hallucination that merged fact with invention. In addition, each cited purported NYT articles that did not exist. The reporters spotted the errors easily because they knew the real answer.
But, what if your business relied on a Generative AI solution to answer questions automatically without human oversight? What if you were relying on it to identify patterns or generate texts that human workers would not be able to fact check? What if using GenAI to create ad or email copy? In these cases, AI hallucinations could pose serious reputational and legal risks to your business.
3 AI hallucinations risks
Every enterprise function is actively exploring how to leverage Generative AI. McKinsey estimates the biggest economic and productivity impacts from the technology will be for marketing, sales, software development, and customer operations. Yet back office functions like HR, finance, legal, procurement, and others are also actively exploring Generative AI applications. The major risks from AI hallucinations span the different functions where the technology is being used, including:
AI hallucinations risk #1—Erosion of brand identity
If AI hallucinations include information that is false, misleading, or in any way inconsistent with the way you speak to your customer, it can erode your brand identity. By extension, they can damage the connection customers have with you.
Many factors go into cultivating a brand image capable of cultivating loyalty. The look and feel of your products and locations, how you deliver your services, and the tone and approach you take with your marketing all contribute to the feeling you want customers to have from working with you. Consistency is key. If your physical stores, branches, or outlets offer one experience and your digital channels another, it can unsettle your customers and make them look elsewhere.
Without strong controls and even human oversight, AI-generated messages could spread inaccurate information about your products or services. They could also make commitments that the brand cannot fulfill without significant expense. That’s one way AI hallucinations create risk for your brand.
Consider AI chatbots commonly used in chatbot marketing, one of the common applications of Generative AI. If those chatbots generate hallucinations at scale promising refunds or bonus products, it could cost a brand either significant loyalty (if it does not honor the promise) or significant revenue (if it does).
AI hallucinations risk #2—Ill-informed decision making
Another popular use case for AI is to automate common decisions to free up human oversight for more complex work. Effective automation depends on accurate information and consistent execution, however. If an algorithm prompts a Generative AI tool to summarize a series of customer interactions and the AI introduces hallucinations, any decisions made based on the summary would be misinformed.
The level of risk this presents varies greatly. Getting the decision about what product to offer a given customer in an email marketing campaign may be relatively low risk, given the average customer engagement rate. In contrast, a decision to erroneously close a financial account could lead to serious reputational damage. The same is true for hallucinations in systems used by your finance department or HR.
AI hallucinations risk #3—Legal or regulatory risk
Law firms have shown high interest in Generative AI, as have the legal departments of enterprises. AI is also making the job of compliance officers easier. It does this by allowing them to scan and generate documents and monitor regulatory changes. With all this promise for improving legal and regulatory compliance, however, come risks. Accuracy is paramount for legal and compliance professionals. AI hallucinations threaten to introduce inaccuracies that are hard to identify and correct, given that they can be buried inside long pages of legalese. If these inaccuracies appear in financial statements of public companies, it could result in legal actions against the firm and its executive officers.
How to limit AI hallucinations
AI experts are not sure why Generative AI tends to hallucinate, though it is a widespread problem. Research on the most popular large language models (LLMs) found that all of the tested systems hallucinated. In a recent study, researchers prompted GPT-4 (OpenAI), Palmchat (Google), Claude 2 (Anthropic) and systems from Meta to summarize text. All of the AI-generated responses included hallucinations, though with varying levels of frequency. The lowest was 3 percent of the GPT-4-generated text up to 27 percent of the Palmchat-generated text. Researchers suspect that the frequency of hallucinations may be higher if the AI is asked to do something more complex and spontaneous than the simple text summarization task used in this study.
There are a number of technological and process approaches that can help reduce hallucinations. They include:
- High training data quality. Data sets composed exclusively from accurate text may generate text with inaccuracies, according to research, though it is less likely.
- Limited training data quantity. Models built on huge language data sets are more likely to hallucinate than those built on more limited data sets. The future of business impact from Generative AI and large language models is by starting small.
- Task specificity. General-purpose Generative AI solutions tend to hallucinate more often than solutions trained to perform a specific task. One of the suspected reasons why is that the general purpose solutions operate by predicting the next likely word in a sentence. That general approach often leaves a lot of options and room for interpretation. Point solutions, in contrast, are solving a specific problem with a narrower goal, which creates more guardrails within which the AI operates. With those constraints, the AI may be less likely to freelance.
- Cross-referencing. Instead of relying on the results from Generative AI, some providers are cross-referencing it with sources that use different methods. Microsoft’s Bing team, for example, leverages GPT-4 to enhance its search and chat capabilities. It combines the results from a Bing Search with a GPT-4 query to reduce the chance that hallucinations appear in chat responses. Think of it as using AI to fact check AI.
- Technical enhancement. A number of methods allow AI engineers to enhance Generative AI models to improve their results, make them more relevant, and mitigate hallucinations. Prompt engineering and fine-tuning are two of the more common and familiar methods. Prompt engineering involves crafting prompts in a narrow and specific way that limits the results. Fine-tuning involves refined training of the AI model either on a specific subset of data or on specific outputs. Another approach is retrieval-augmented generation (RAG). This augments a given LLM with information from another knowledge base with the goal of making its responses more accurate, relevant, or up-to-date.
- Human involvement in model learning. Humans are and will long continue to be key to the development of useful and effective AI solutions. It is humans, after all, who tell the AI whether its answer is consistent with the prompt, helpful, and accurate. Human oversight will be an essential aspect of any AI risk management approach (even with the acknowledgement that some AI solutions are intended to overcome the biases in human decision making).
Persado Motivation AI and hallucinations
At Persado, we have been working for more than ten years to enhance our customers’ relationships using language. Our enterprise Generative AI is built on a proprietary language model that includes more than 10 years of marketing messages from Fortune 500 companies. Our focus on generating the marketing copy that is most likely to motivate customer action gives our text generation and machine learning technology a focused goal of improving your campaign results by optimizing for conversions. The Persado content intelligence team—composed of human writers, linguists, and data analysts—also works with the model to teach it what “good” language looks like and steer it away from inauthentic, off-brand, or inaccurate options.
In short, our team leverages a specific pool of high-quality data, coupled with a focused task and human oversight, to generate language that increases customer engagement above human-generated controls 96% of the time. The result is better results and higher customer engagement with no AI hallucination risk.
Learn more about Persado Motivation AI and explore getting your risk-free trial today.